Breadcrumb
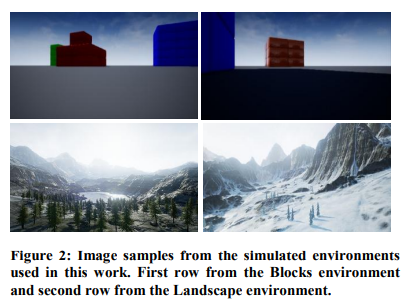
Deep convolutional neural network based autonomous drone navigation
This paper presents a novel approach for aerial drone autonomous navigation along predetermined paths using only visual input form an onboard camera and without reliance on a Global Positioning System (GPS). It is based on using a deep Convolutional Neural Network (CNN) combined with a regressor to output the drone steering commands. Furthermore, multiple auxiliary navigation paths that form a â€n˜avigation envelope' are used for data augmentation to make the system adaptable to real-life deployment scenarios. The approach is suitable for automating drone navigation in applications that
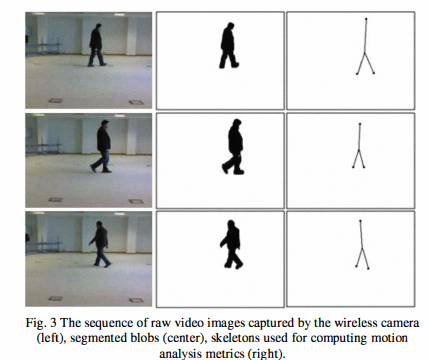
Ambient and wearable sensing for gait classification in pervasive healthcare environments
Pervasive healthcare environments provide an effective solution for monitoring the wellbeing of the elderly where the general trend of an increasingly ageing population has placed significant burdens on current healthcare systems. An important pervasive healthcare system functionality is patient motion analysis where gait information can be used to detect walking behavior abnormalities that may indicate the onset of adverse health problems, for quantifying post-operative recovery, and to observe the progression of neurodegenerative diseases. The development of accurate motion analysis models
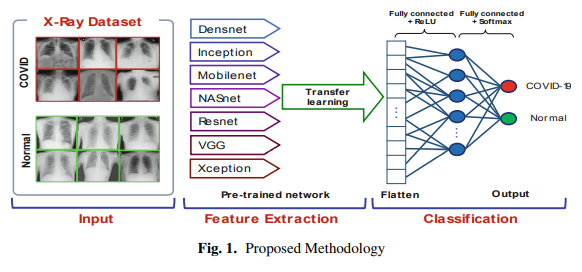
Detection of COVID-19 from Chest X-Ray Images Using Deep Neural Network with Fine-Tuning Approach
The coronavirus (COVID-2019) quickly spread throughout the world and came to be a pandemic. To avoid further spreading this epidemic and treat affected patients rapidly, it is important to recognize the positive cases as early as possible. In this paper, deep learning techniques are employed to detect COVID-19 from chest X-ray images quickly. The images of the two classes, COVID and No-findings are collected from three public datasets. The proposed approach consists of two phases; transfer learning and fine-tuning. Transfer learning is carried out by seven deep learning models: DenseNet
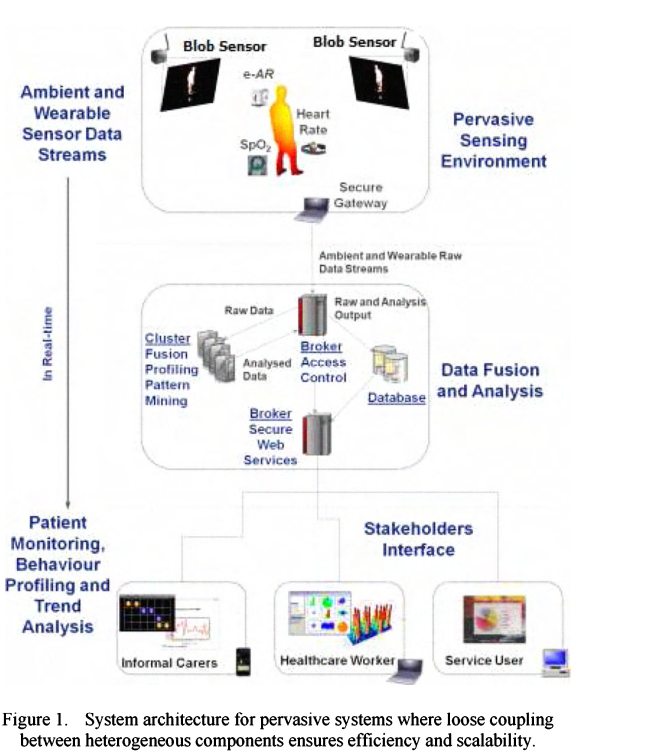
An integrated multi-sensing framework for pervasive healthcare monitoring
Pervasive healthcare provides an effective solution for monitoring the wellbeing of elderly, quantifying post-operative patient recovery and monitoring the progression of neurodegenerative diseases such as Parkinson's. However, developing functional pervasive systems is a complex task that entails the creation of appropriate sensing platforms, integration of versatile technologies for data stream management and development of elaborate data analysis techniques. This paper describes a complete and an integrated multi-sensing framework, with which the sensing platforms, data fusion and analysis
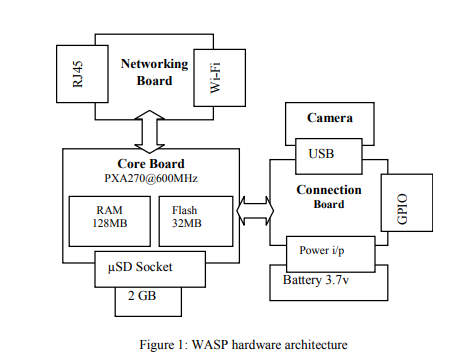
WASP: Wireless autonomous sensor prototype for Visual Sensor Networks
Visual Sensor Networks (VSNs) enable enhanced three-dimensional sensing of spaces and objects, and facilitate collaborative reasoning to open up a new realm of vision-based distributed smart applications including security/surveillance, healthcare delivery, traffic monitoring, just to name a few. However, such applications require sensor nodes that can efficiently process large volumes of visual information in-situ and exhibit intelligent behavior to support autonomous operation, scalability, and energy efficiency. This paper presents WASP, a vision sensor node prototype with high
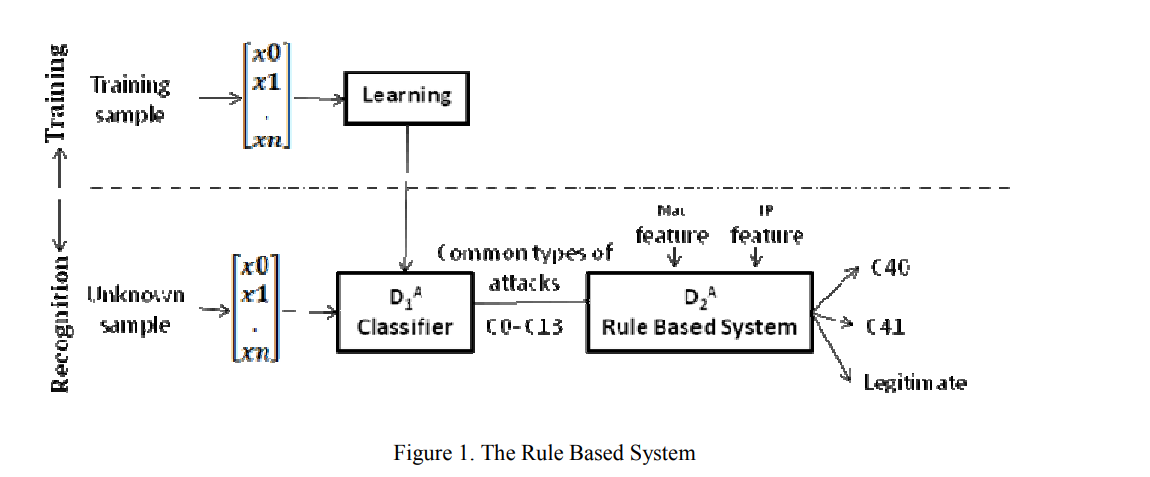
Misfeasor classification and detection models using machine learning techniques
Misfeasors (or insiders) are considered among the most difficult intruders to detect due to their knowledge and authorization within the organization. Machine learning techniques have been widely used for intrusion detection but only little work has addressed the use of machine learning for detecting and classifying different types of insiders. The aim of this study is to exploit different recognition models for misfeasors detection by adding the Mac address as a feature in classification. Three different recognition models (a Rule Based Model, a Hierarchical Classification Model and a
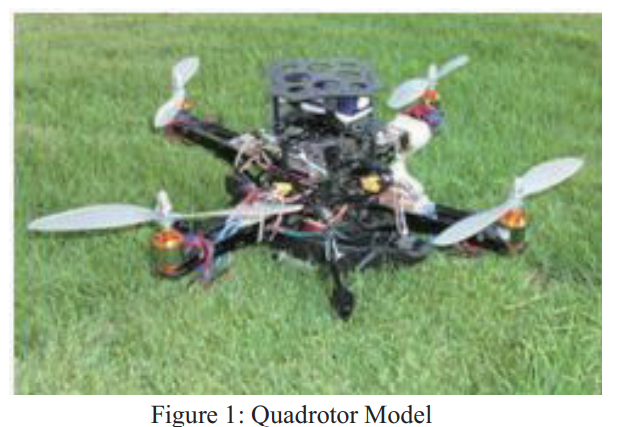
On-board multiple target detection and tracking on camera-equipped aerial vehicles
This paper presents a novel automatic multiple moving target detection and tracking framework that executes in real-time with enhanced accuracy and is suitable for UAV imagery. The framework is deployed for on-board processing and tested over datasets collected by our UAV system. The framework is based on image feature processing and projective geometry and is carried out on the following stages. First, FAST corners are detected and matched, and then outlier features are computed with least median square estimation. Moving targets are subsequently detected by using a density-based spatial
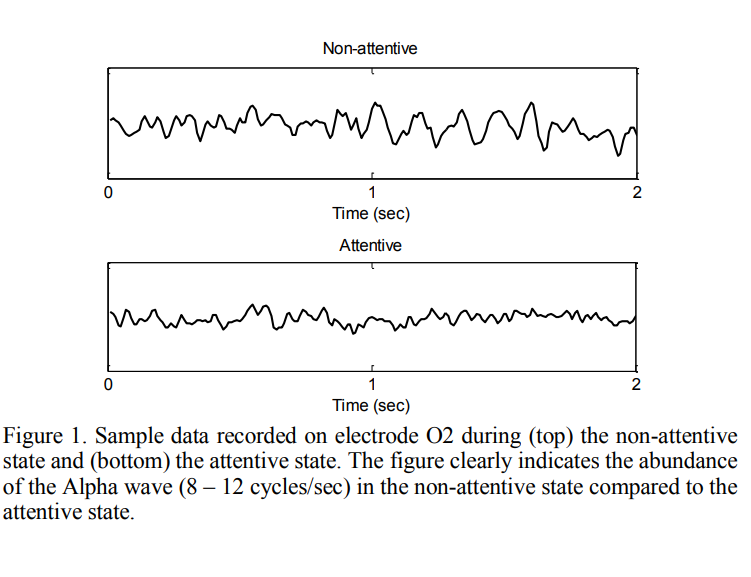
EEG spectral analysis for attention state assessment: Graphical versus classical classification techniques
Advances in Brain-computer Interface (BCI) technology have opened the door to assisting millions of people worldwide with disabilities. In this work, we focus on assessing brain attention state that could be used to selectively run an application on a hand-held device. We examine different classification techniques to assess brain attention state. Spectral analysis of the recorded EEG activity was performed to compute the Alpha band power for different subjects during attentive and non-attentive tasks. The estimated power values were used to train a number of classical classifiers to
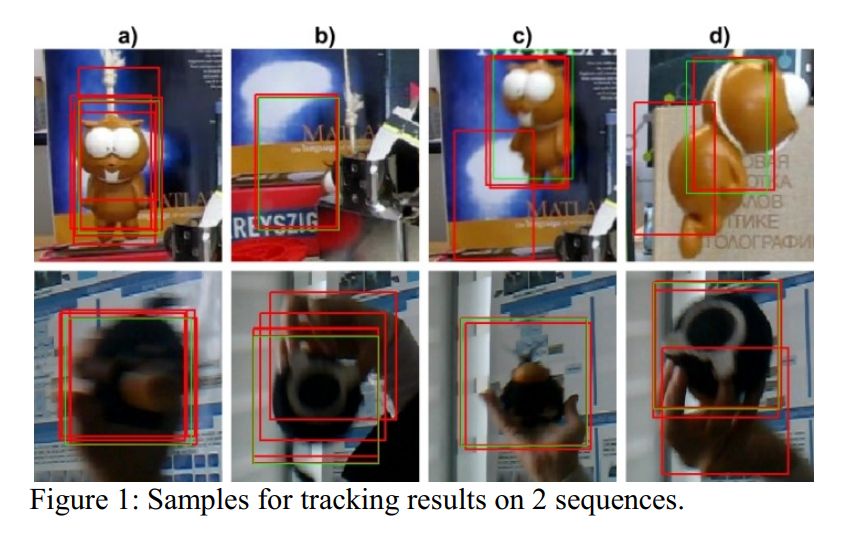
Robust real-time tracking with diverse ensembles and random projections
Tracking by detection techniques have recently been gaining popularity and showing promising results. They use samples classified in previous frames to detect an object in a new frame. However, because they rely on self updating, such techniques are prone to object drift. Multiple classifier systems can be used to improve the detection over that of a single classifier. However, such techniques can be slow as they combine information from different tracking methods. In this paper we propose a novel real-time ensemble approach to tracking by detection. We create a diverse ensemble using random
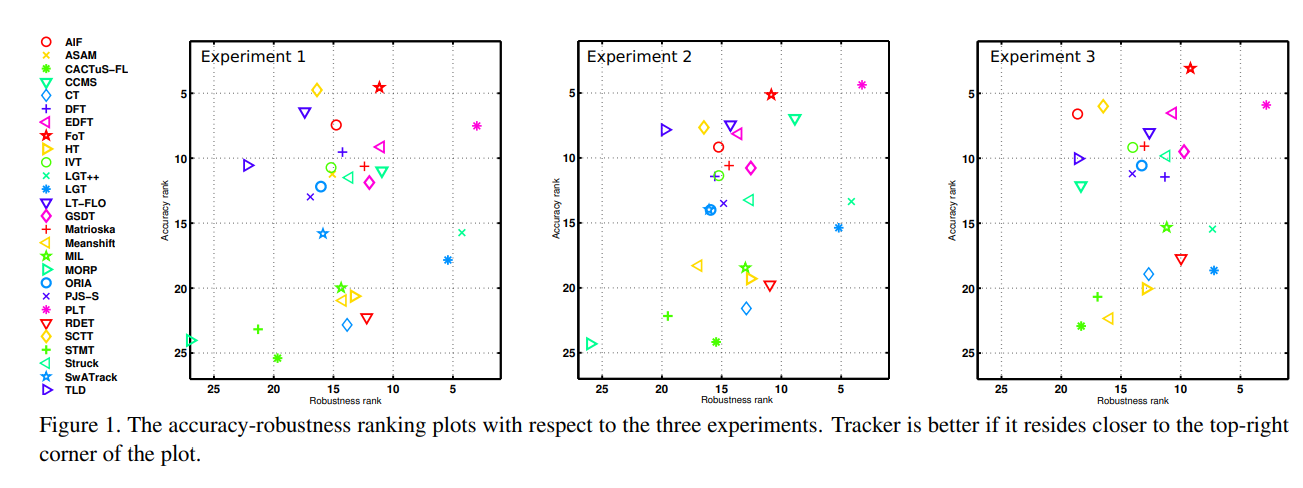
The visual object tracking VOT2013 challenge results
Visual tracking has attracted a significant attention in the last few decades. The recent surge in the number of publications on tracking-related problems have made it almost impossible to follow the developments in the field. One of the reasons is that there is a lack of commonly accepted annotated data-sets and standardized evaluation protocols that would allow objective comparison of different tracking methods. To address this issue, the Visual Object Tracking (VOT) workshop was organized in conjunction with ICCV2013. Researchers from academia as well as industry were invited to participate
Pagination
- Previous page ‹‹
- Page 27
- Next page ››