Breadcrumb
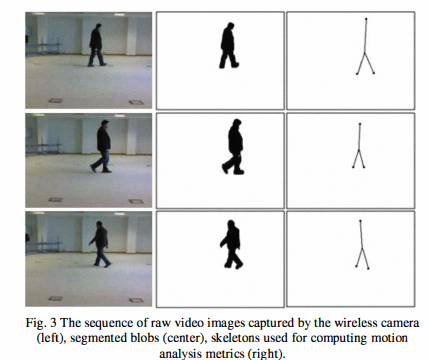
Ambient and wearable sensing for gait classification in pervasive healthcare environments
Pervasive healthcare environments provide an effective solution for monitoring the wellbeing of the elderly where the general trend of an increasingly ageing population has placed significant burdens on current healthcare systems. An important pervasive healthcare system functionality is patient motion analysis where gait information can be used to detect walking behavior abnormalities that may indicate the onset of adverse health problems, for quantifying post-operative recovery, and to observe the progression of neurodegenerative diseases. The development of accurate motion analysis models, however, requires the integration of multi-sensing modalities and the utilization of appropriate data analysis techniques. This paper describes a simple and robust framework for improved patient motion analysis based on an ambient and a wearable sensor. Using visual information from a single vision sensor, target segmentation is first carried out and a skeleton extraction procedure is subsequently applied to quantify the target internal motion by computing two metrics, spatiotemporal cyclic motion between leg segments and head trajectory. Extracted accelerometer information from a wearable body sensor is fused with the extracted metrics at the feature level by using K-Nearest Neighbor algorithm to classify target's walking gait into normal or abnormal. The potential value of the proposed framework for patient monitoring is demonstrated and the results obtained from practical experiments are described. © 2010 IEEE.