Breadcrumb
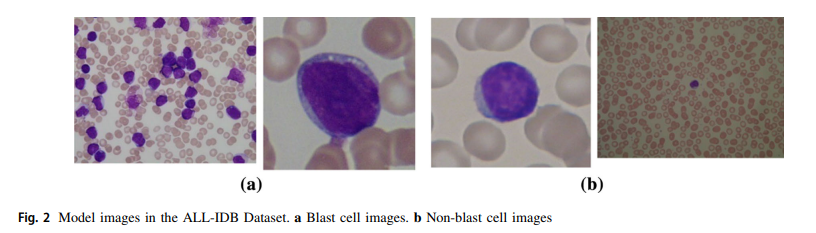
Rough set theory with Jaya optimization for acute lymphoblastic leukemia classification
Early diagnosis of malignant leukemia can enormously help the physicians in choosing the right treatment for the patient. A lot of diagnostic techniques are available to identify leukemia disease, but these techniques are costly. Hence, there is a need for a less time-consuming and cost-effective method for the classification of leukemia blood cells. In this paper, application of graphical user interface technique for the differentiation of acute lymphoblastic leukemia nucleus from healthy lymphocytes in a medical image is described. This method employs backtrack search optimization algorithm
The design of x-ray film reader with film presence detector
X-Ray viewer is a tool for observing the results of X-Ray films using ray lighting. It aims to get clearer readings of X-Ray films by radiographers and doctors. X-Ray viewers in hospitals generally cannot be carried anywhere because they use fluorescent lamps as a source of radiation and use 220 Volt AC voltage directly. So that its use is less effective and efficient because it must be connected directly to a 220 Volt AC power source and requires large power. In this regard the author wants to design an X-Ray viewer tool that can be used to read the results of X-Ray films clearly and is
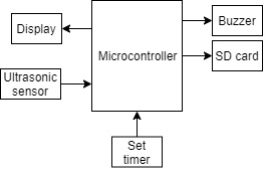
Simulation of vitiligo therapy equipment
Vitiligo is a skin disorder caused by a lack of melanin pigment in the skin, which causes white patches on certain parts of the skin because this melanin pigment is not able to produce the skin color. Previously, one of the treatments for vitiligo was using a UVB lamp with a 311 nm wavelength that could not yet be adjusted to dim the lights as safety when conducting therapy. Therefore, the research aims to design a simulation of the vitiligo therapy device equipped with a timer LED lamp, a safety of lighting, and the data storage. The data are stored in the SD Card to make it easier for
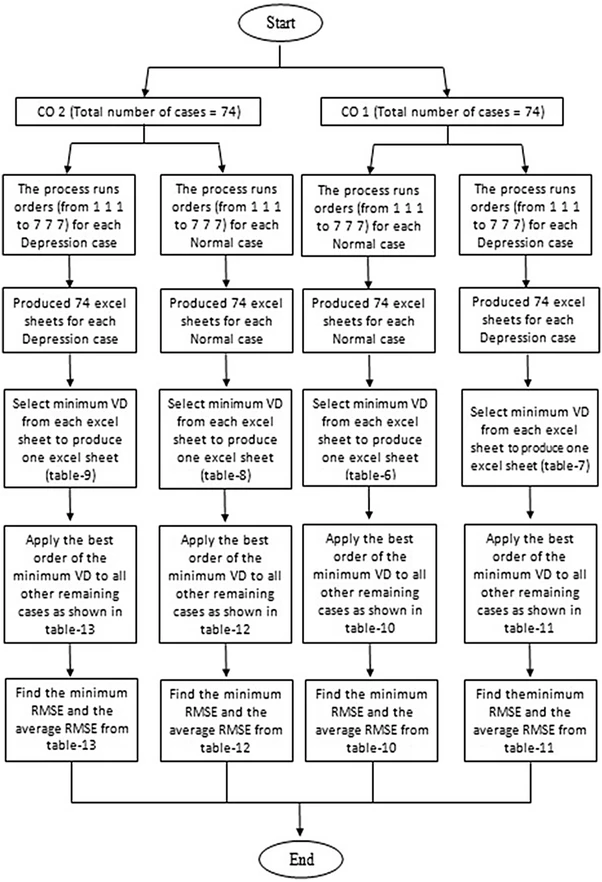
Nonlinear single-input single-output model-based estimation of cardiac output for normal and depressed cases
Mental depression is associated with an increased risk of cardiovascular mortality, thus provisioning generic simple nonlinear mathematical models for normal and depressed cases using only heart rate (HR) or stroke volume (SV) as a single input to produce cardiac output (CO) as a single output instead of using both HR and SV as two inputs. The proposed models could be in the future an effective tool to investigate the effect of neuroleptic medication, especially depression, and it reduces the time of processing. Seventy-four depressed cases, 74 normal peers and autoregressive considered as a
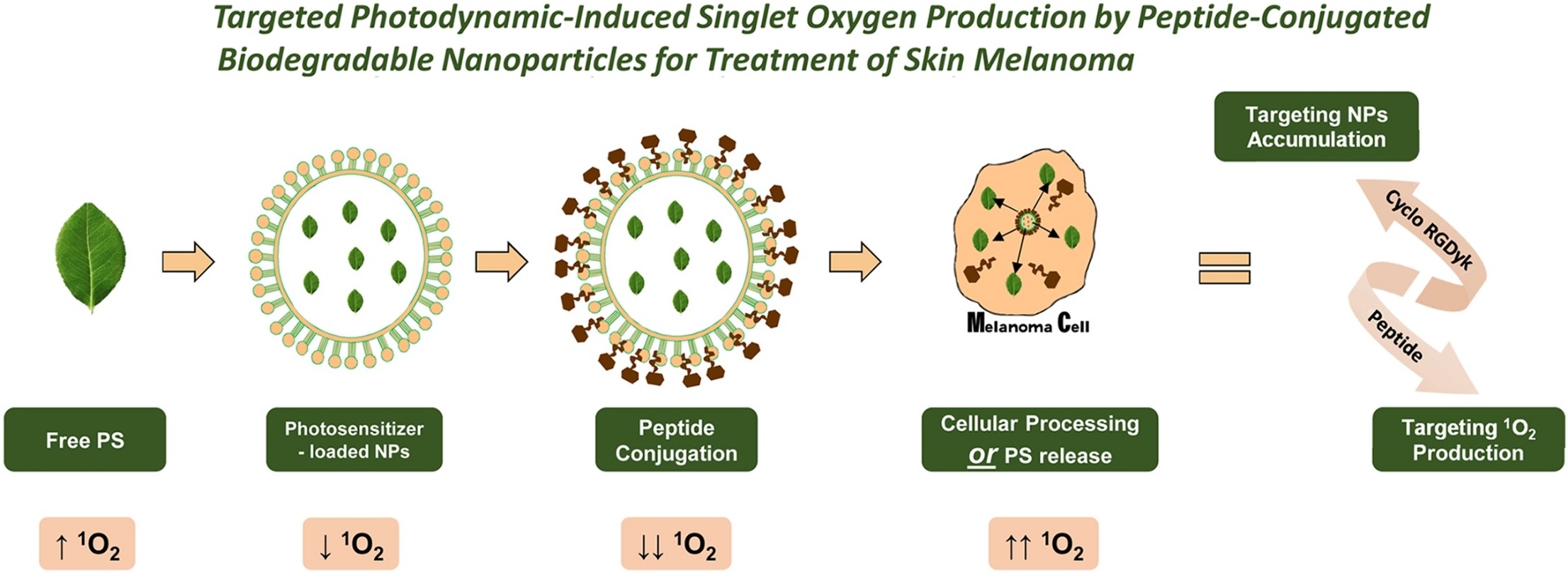
Targeted photodynamic-induced singlet oxygen production by peptide-conjugated biodegradable nanoparticles for treatment of skin melanoma
Background: Photodynamic therapy (PDT) has been determined to be a promising treatment modality in the most resistant tumors such as malignant melanoma. However, the key cytotoxic agent of PDT, -singlet oxygen (1O2) - represents a high risk of photodynamic-associated side effects e.g. skin photosensitization. Recently, controllable photosensitization, where 1O2 is produced on demand, has received increasing attention. In our study, this could be achieved via loading the photosensitizer (PS) in nanoparticles (NPs) decorated with target-specific moieties characterized by 1O2 quenching abilities
Combined effect of wind speed and covering irrigation canals on water quality parameters
Wind has a considerable effect on many water quality parameters. Some of the parameters are directly affected by the wind, while others are influenced by other physical water parameters like the velocity, temperature. etc.That are affected by wind and hence transfer their effect to water quality parameters. As the wind has an effect on water quality parameters, also covering waterways has a great effect on the water quality of those covered waterways. This is because covering a waterway alters the concentrations of its water quality parameters. This research is concerned with studying the
Combined effect of wind speed and covering irrigation canals on water quality parameters
Wind has a considerable effect on many water quality parameters. Some of the parameters are directly affected by the wind, while others are influenced by other physical water parameters like the velocity, temperature. etc. that are affected by wind and hence transfer their effect to water quality parameters. As the wind has an effect on water quality parameters, also covering waterways has a great effect on the water quality of those covered waterways. This is because covering a waterway alters the concentrations of its water quality parameters. This research is concerned with studying the
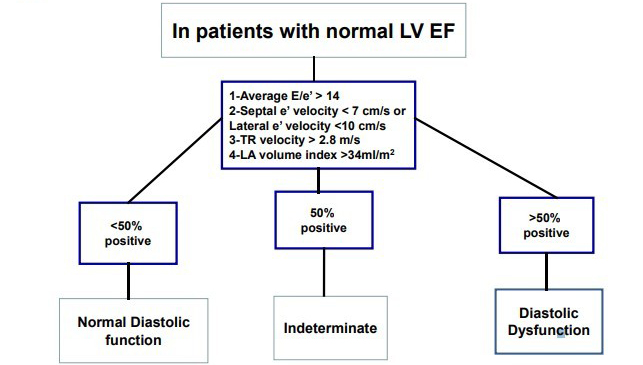
Left ventricular diastolic function in type 2 diabetes mellitus is associated with myocardial triglyceride content but not with impaired myocardial perfusion reserve
Purpose: To study myocardial perfusion reserve and myocellular metabolic alterations indicated by triglyceride content as possible causes of diastolic dysfunction in patients with type 2 diabetes mellitus, preserved systolic function, and without clinically evident coronary artery disease. Materials and Methods: Patients with type 2 diabetes mellitus (n = 42) underwent cardiac magnetic resonance (CMR) for quantification of 1) myocardial contractility by strain-encoded MR (SENC); 2) myocardial triglyceride content by proton magnetic resonance spectroscopy ( 1H-MRS); and 3) myocardial perfusion
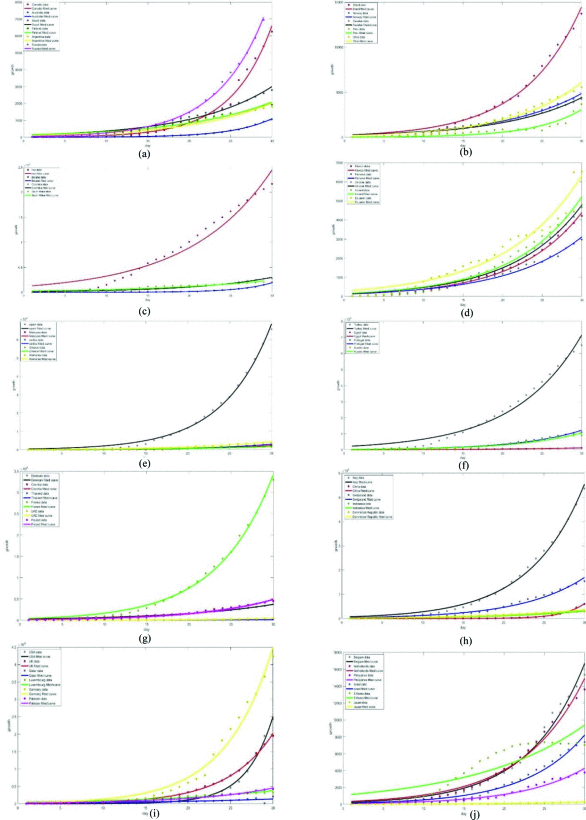
Modeling the effect of population density on controlling Covid-19 initial Spread with the use of MATLAB numerical methods and stringency index model
The effect of population density on the initial spread of the novel Covid-19 virus has been evaluated using the numerical data of fifty pioneer adopting countries in their first thirty days experience with the disease. The fifty countries were curdled into ten groups that each of them possesses an average population density and each group's virus's spread was modeled in a two-dimensional graph with the use of MATLAB curve fitting. The modeling is done based on the exponential growth equation. The stringency index model was also utilized a source of analysis regarding the government responses
Pagination
- Previous page ‹‹
- Page 13
- Next page ››