Breadcrumb
Convolutional Neural Network with Attention Modules for Pneumonia Detection
In 2017, pneumonia was the primary diagnosis for 1.3 million visits to the Emergency Department (ED) in the United States. The mortality rate was estimated to be 5%-10% of hospitalized patients, whereas it rises to 30% for severe cases admitted to the Intensive Care Unit (ICU). Among all cases admitted to ED, 30% were misdiagnosed, and they did not suffer from pneumonia, which raises a flag for the need for more accurate diagnosis methods. Several methods for pneumonia detection were recently developed using AI in general and more specifically, using deep neural networks. Even though it worth
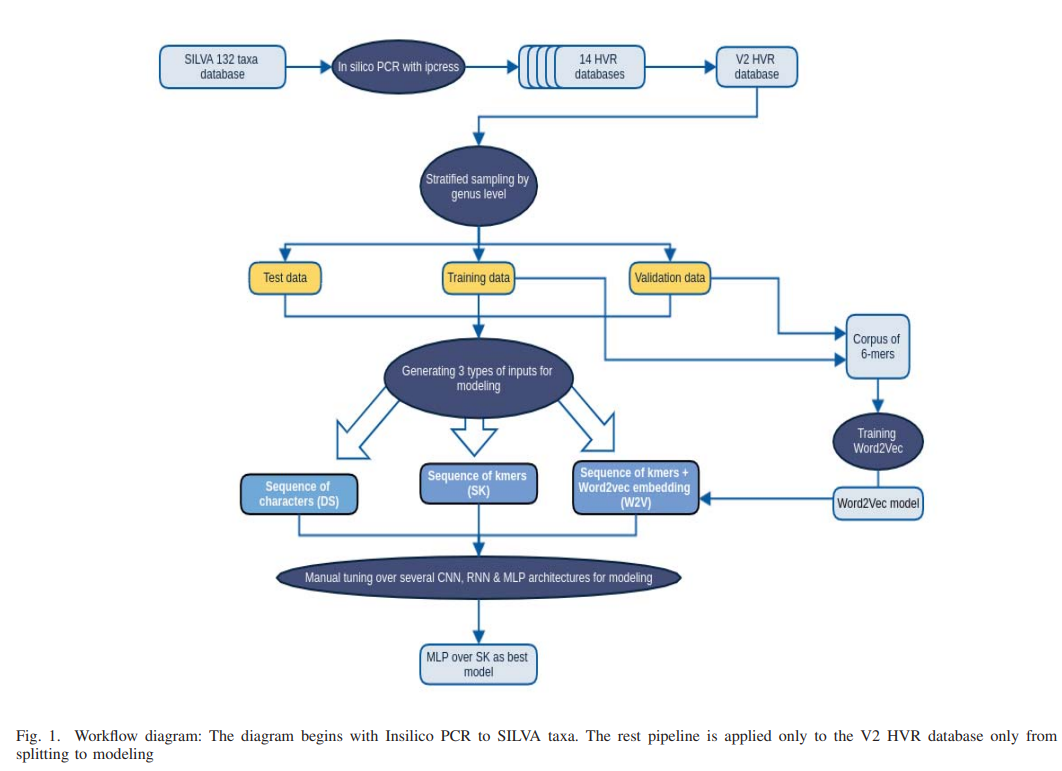
AmpliconNet: Sequence Based Multi-layer Perceptron for Amplicon Read Classification Using Real-time Data Augmentation
Taxonomic assignment is the core of targeted metagenomics approaches that aims to assign sequencing reads to their corresponding taxonomy. Sequence similarity searching and machine learning (ML) are two commonly used approaches for taxonomic assignment based on the 16S rRNA. Similarity based approaches require high computation resources, while ML approaches dont need these resources in prediction. The majority of these ML approaches depend on k-mer frequency rather than direct sequence, which leads to low accuracy on short reads as k-mer frequency doesnt consider k-mer position. Moreover
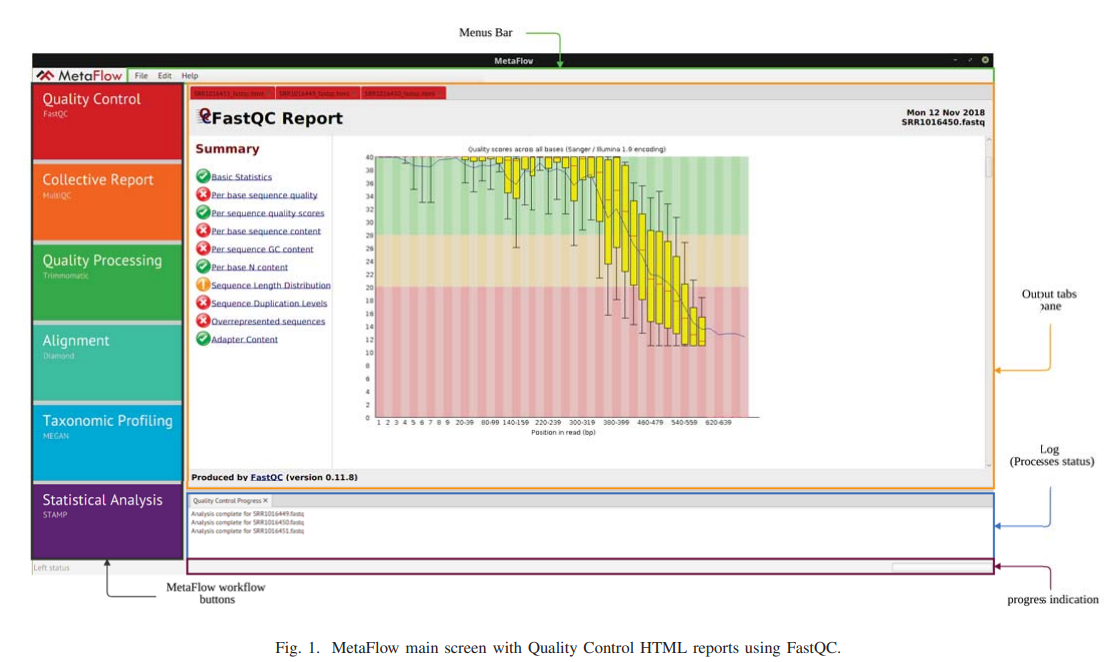
MetaFlow: An interactive user-friendly workflow for automated analysis of whole genome shotgun sequencing metagenomic data
Metagenomics is a rapidly emerging field that is concerned with the study of microbial communities 'microbiomes' on both levels of taxonomic classification and functional annotation. Targeted amplicon (16S rRNA) and whole genome shotgun (WGS) sequencing are the two main sequencing strategies in metagenomics. As amplicon sequencing provides a cheap way to classify the composition of a microbial community, it lacks the ability to identify microbial genes and annotate its corresponding functions. On the other hand, WGS sequencing allows further investigation of the complete genomes with all
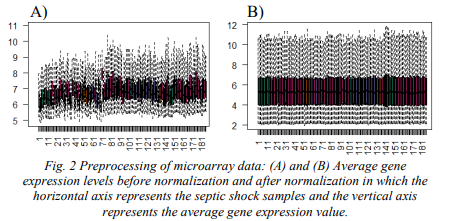
Studying Genes Related to the Survival Rate of Pediatric Septic Shock
Pediatric septic shock is generally considered as a devastating clinical syndrome that can lead to tissue damage and organ failure due to the over exaggerated immune response to an infection. Therefore, in this paper, we attempted to early identify the clinical course of such disease with the aid of peripheral blood T-cells of 181 pediatric patients who admitted to Intensive Care Unit (ICU), Accordingly, 34 differential expressed genes have been identified as biological genetic biomarkers. Minimum redundancy and maximum relevance feature selection strategy has been proposed for the discovery
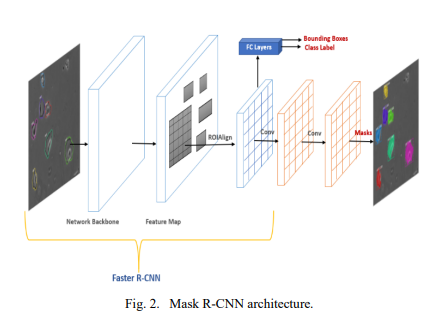
Instance Segmentation of 2D Label-Free Microscopic Images using Deep Learning
The precise detection and segmentation of cells in microscopic image sequences is an essential task in biomedical research, such as drug discovery and studying the development of tissues, organs, or entire organisms. However, the detection and segmentation of cells in phase contrast images with a halo and shade-off effects is still challenging. Lately, Mask Regional Convolutional Neural Network (Mask R-CNN) has been introduced for object detection and instance segmentation of natural images. This study investigates the efficacy of the Mask R-CNN to instantly detect and segment label-free
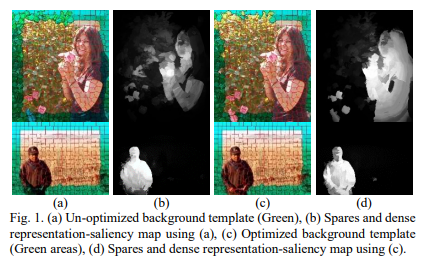
Robust Background Template for Saliency Detection
In this paper, we propose an effective saliency detection method based on dense and sparse representation in-terms of an optimized background template. Firstly, the input image is divided into compact and uniform super-pixels. Then, the optimized background template is produced by introducing boundary conductivity measurement to improve the dense and sparse representation of the image's super-pixels in terms of the optimized background, where the reconstruction error represents a saliency measure. Based on the optimized template, two saliency maps are generated by dense and sparse
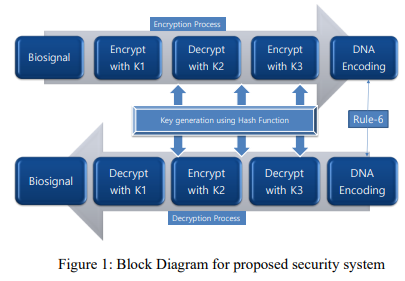
An E-health System for Encrypting Biosignals Using Triple-DES and Hash Function
This Electronic Health (E-Health) is a broad expression that enables the communication between healthcare professionals in handling patient information through the cloud. Exchanging medical data over the public cloud requires securing transferring for the data that's direct many researchers in proposing different secure schemes to enable users to handle data safely without hacking or alternating. In this study, one of the most common encryption algorithms called Triple Data Encryption Standard (Triple-DES) has been implemented with the aid of the hash function and DNA cryptography base to
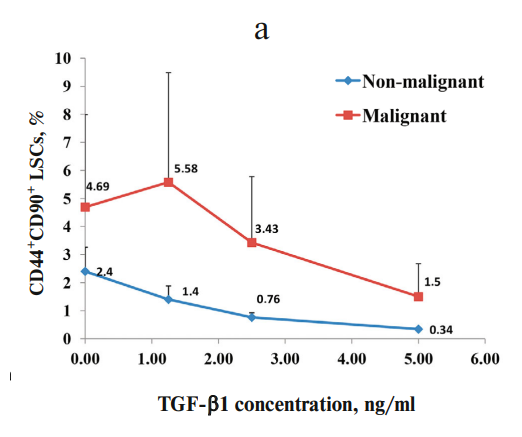
Role of TGF-β1 and C-Kit Mutations in the Development of Hepatocellular Carcinoma in Hepatitis C Virus-Infected Patients: in vitro Study
Transforming growth factor beta (TGF-β) acts as a tumor-suppressing cytokine in healthy tissues and non-malignant tumors. Yet, in malignancy, TGF-β can exert the opposite effects that can promote proliferation of cancer cells. C-Kit plays a prominent role in stem cell activation and liver regeneration after injury. However, little is known about the cross-talk between TGF-β and C-Kit and its role in the progression of hepatocellular carcinoma (HCC). Here, we studied the effect of increasing doses of TGF-β1 on CD44+CD90+ liver stem cells (LSCs) and C-Kit gene expression in malignant and
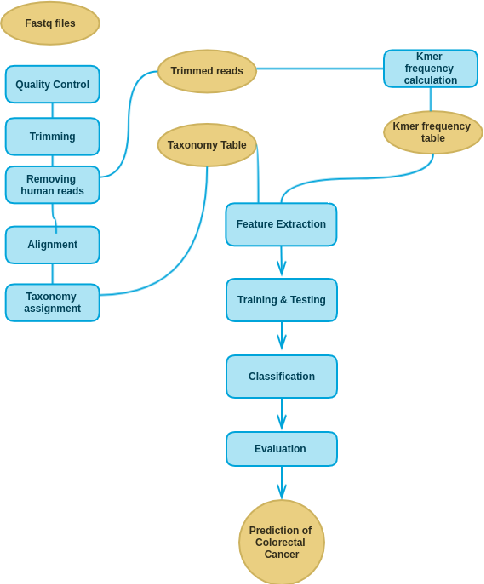
A Hybrid Machine Learning Approach for the Phenotypic Classification of Metagenomic Colon Cancer Reads Based on Kmer Frequency and Biomarker Profiling
Human Microbiome plays a critical role in health and the environment. Colorectal cancer (CRC) is the most common cause of death in many countries, and hence early diagnosis of CRC may help in increasing the survival rate. Tracking changes in the microbiome structure of human gut opens new gates towards the detection and prediction of the risk of CRC. Recently, machine learning became a powerful technique in many bioinformatics fields, one of which is metagenomics. Metagenomics is defined as the study of a collection of microbial genomes isolated directly and sequenced from its natural habitats
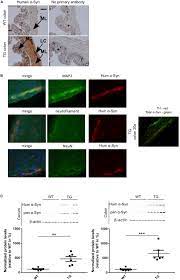
Enriched environmental conditions modify the gut microbiome composition and fecal markers of inflammation in parkinson’s disease
Recent findings suggest an implication of the gut microbiome in Parkinson’s disease (PD) patients. PD onset and progression has also been linked with various environmental factors such as physical activity, exposure to pesticides, head injury, nicotine, and dietary factors. In this study, we used a mouse model, overexpressing the complete human SNCA gene (SNCA-TG mice) modeling familial and sporadic forms of PD to study whether environmental conditions such as standard vs. enriched environment changes the gut microbiome and influences disease progression. We performed 16S rRNA DNA sequencing
Pagination
- Previous page ‹‹
- Page 31
- Next page ››