Breadcrumb
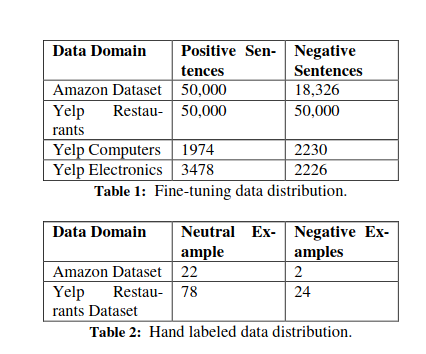
NileTMRG at SemEval-2016 task 5: Deep convolutional neural networks for aspect category and sentiment extraction
This paper describes our participation in the SemEval-2016 task 5, Aspect Based Sentiment Analysis (ABSA). We participated in two slots in the sentence level ABSA (Subtask 1) namely: aspect category extraction (Slot 1) and sentiment polarity extraction (Slot 3) in English Restaurants and Laptops reviews. For Slot 1, we applied different models for each domain. In the restaurants domain, we used an ensemble classifier for each aspect which is a combination of a Convolutional Neural Network (CNN) classifier initialized with pretrained word vectors, and a Support Vector Machine (SVM) classifier
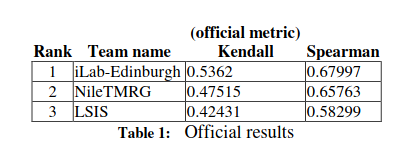
NileTMRG at SemEval-2016 Task 7: Deriving prior polarities for Arabic sentiment terms
This paper presents a model that was developed to address SemEval Task 7: "Determining Sentiment Intensity of English and Arabic Phrases", with focus on 'Arabic Phrases'. The goal of this task is to determine the degree to which some given term is associated with positive sentiment. The underlying premise behind the model that we have adopted is that determining the context (positive or negative) in which a term usually occurs can determine its strength. Since the focus is on Twitter terms, Twitter was used to collect tweets for each term for which a strength value was to be derived. An Arabic
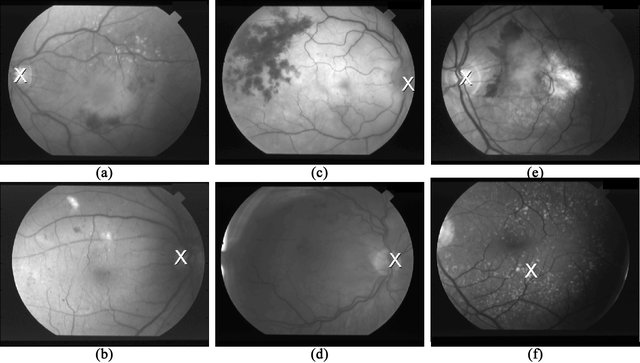
Ultrafast optic disc localization using projection of image features
Optic Disc (OD) localization is a fundamental step in developing computer-assisted diagnostics. In this work, an ultrafast method to locate the OD in retinal fundus images is presented. The proposed method is based on transforming the localization problem into two 1D problems by projecting the image features onto two perpendicular directions. Image features such as the directionality of the retinal vessels, the brightness and the size of the OD have been used in the current method. Two publicly available databases were used to evaluate the accuracy and the computation time of the proposed
MLP, gaussian processes and negative correlation learning for time series prediction
Time series forecasting is a challenging problem, that has a wide variety of application domains such as in engineering, environment, finance and others. When confronted with a time series forecasting application, typically a number of different forecasting models are tested and the best one is considered. Alternatively, instead of choosing the single best method, a wiser action could be to choose a group of the best models and then to combine their forecasts. In this study we propose a combined model consisting of Multi-layer perceptron (MLP), Gaussian Processes Regression (GPR) and a
Predicting all star player in the national basketball association using random forest
National Basketball Association (NBA) All Star Game is a demonstration game played between the selected Western and Eastern conference players. The selection of players for the NBA All Star game purely depends on votes. The fans and coaches vote for the players and decide who is going to make the All Star roster. A player who continues to receive enough votes in following years will play more All Star games. The selection of All Star players in NBA is subjective based on voting and there are no selection criteria that take out the human bias and opinion. Analyzing data from previous sports
Emotions analysis of speech for call classification
Most existing research in the area of emotions recognition has focused on short segments or utterances of speech. In this paper we propose a machine learning system for classifying the overall sentiment of long conversations as being Positive or Negative. Our system has three main phases, first it divides a call into short segments, second it applies machine learning to recognize the emotion for each segment, and finally it learns a binary classifier that takes the recognized emotions of individual segments as features. We investigate different approaches for this final phase by varying how
A novel segmentation method to identify left ventricular infarction in short-axis composite strain-encoded magnetic resonance images
Composite Strain Encoding (CSENC) is a new Magnetic Resonance Imaging (MRI) technique for simultaneously acquiring cardiac functional and viability images. It combines the use of Delayed Enhancement (DE) and the Strain Encoding (SENC) imaging techniques to identify the infracted (dead) tissue and to image the myocardial deformation inside the heart muscle. In this work, a new unsupervised segmentation method is proposed to identify infarcted left ventricular tissue in the images provided by CSENC MRI. The proposed method is based on the sequential application of Bayesian classifier, Otsu's
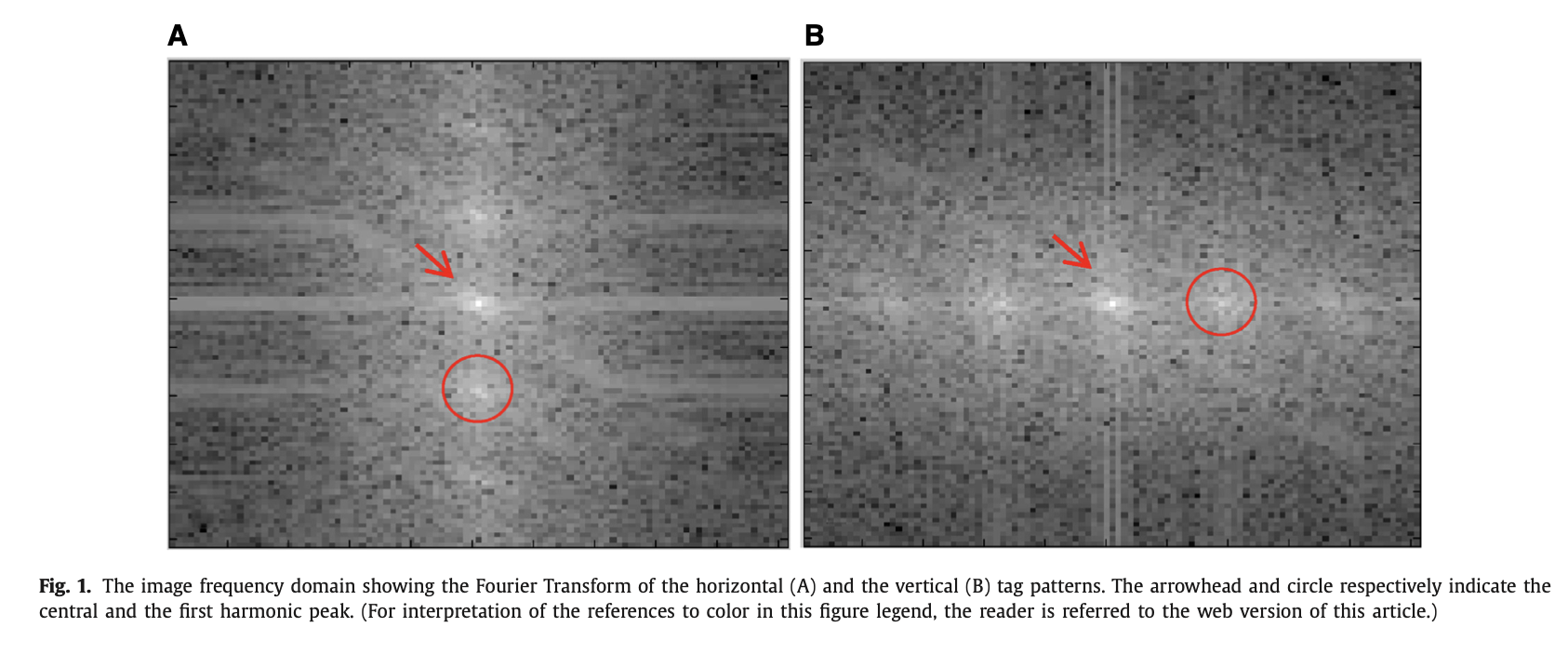
Accurate harmonic phase tracking of tagged MRI using locally-uniform myocardium displacement constraint
Harmonic phase (HARP) tracking is one of the most commonly used techniques for estimating the myocardium regional function from tagged cardiac Magnetic Resonance Imaging sequences. Nevertheless, tag fading and phase distortion can severely limit the tracking accuracy of the technique. In this work, we propose to modify the HARP tracking algorithm to impose a constraint of locally uniform displacement field while tracking the different myocardium points. A numerical contracting phantom and a dataset of 11 patients are used to study the performance of the proposed technique at the different
Efficient distributed computation of maximal exact matches
Given two long strings S and T, representing two genomic sequences, and given a user defined threshold ℓ, the problem of computing maximal exact matches (MEMs) is to find each triple (p 1,p 2,l) specifying two matching substrings S[p 1..p 1 + l - 1] = T[p 2..p 2 + l - 1], such that l ≥ ℓ and S[p 1 - 1] ≠ T[p 2 - 1] and S[p 1 + l] ≠ T[p 2 + l]. Computing MEMs is a major problem in bioinformitcs, because it is a primary step in identifying regions of common similarity among genomic sequences. Faster solutions to this problem are still demanded to overcome the ever increasing amount of genomic
Practical distributed computation of maximal exact matches in the cloud
Computation of maximal exact matches (MEMs) is an important problem in comparing genomic sequences. Optimal sequential algorithms for computing MEMs have been already introduced and integrated in a number of software tools. To cope with large data and exploit new computing paradigms like cloud computing, it is important to develop efficient and ready-to-use solutions running on distributed parallel architecture. In a previous work, we have introduced a distributed algorithm running on a computer cluster for computing the MEMs. In this paper, we extend this work in two directions: First, we
Pagination
- Previous page ‹‹
- Page 16
- Next page ››