Breadcrumb
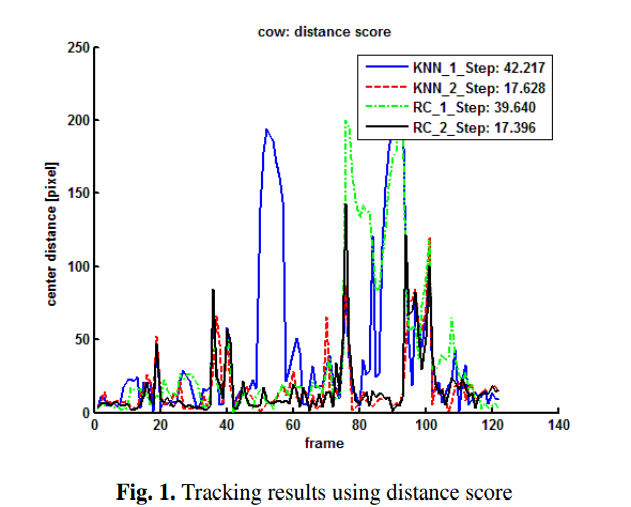
Robust scale-invariant object tracking
Tracking by detection methods are becoming increasingly popular in recent years. They use samples classified in previous frames to detect object in a new frame. These methods have shown successful results. However, due to the self updating nature of this approach, tracking by detection methods usually suffer from object drift. Inaccurately detected samples are added to the training set which degrades the performance. Another problem is that the object may change in shape and size which increases the potential for inaccurate detection and subsequently the chance of losing the object. We propose a robust object tracking algorithm that adapts to changes in the size of the object. The algorithm is divided into two steps. The first step uses random projections compressed using a sparse matrix to describe positive and negative samples generated around the object. These samples are used to train a classifier to detect the position of the object in the next frame. The second step searches for the best size to fit the object around the position chosen in the first step. Experimental results show that our method provides robust tracking and help alleviate the drift problems. © Springer International Publishing Switzerland 2014.