Breadcrumb
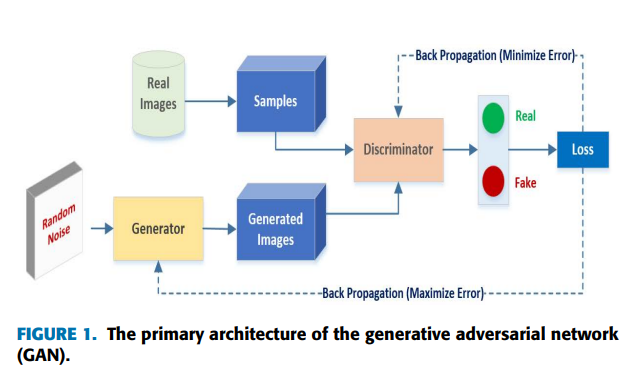
License Plate Image Analysis Empowered by Generative Adversarial Neural Networks (GANs)
Although the majority of existing License Plate (LP) recognition techniques have significant improvements in accuracy, they are still limited to ideal situations in which training data is correctly annotated with restricted scenarios. Moreover, images or videos are frequently used in monitoring systems that have Low Resolution (LR) quality. In this work, the problem of LP detection in digital images is addressed in the images of a naturalistic environment. Single-stage character segmentation and recognition are combined with adversarial Super-Resolution (SR) approaches to improve the quality of the LP by processing the LR images into High-Resolution (HR) images. This work proposes effective changes to the SRGAN network regarding the number of layers, an activation function, and the appropriate loss regularization using Total Variation (TV) loss. The main paper contribution can be summarized into presenting an end-to-end deep learning framework based on generative adversarial networks (GAN), which is able to generate realistic super-resolution images. Also, proposed adding a TV regularization to the loss function to help the model enhance the resolution of images. The proposed SRGAN can handle tiny $72\times 72$ images of LPs. The paper explores how SRGAN performed over different datasets from many aspects, such as visual analysis, PSNR, SSIM, and Optical Character Recognition (OCR). The experiments demonstrate that the suggested SRGAN can generate high-resolution images that improve the accuracy of the license plate recognition stage compared to other systems. © 2013 IEEE.