Breadcrumb
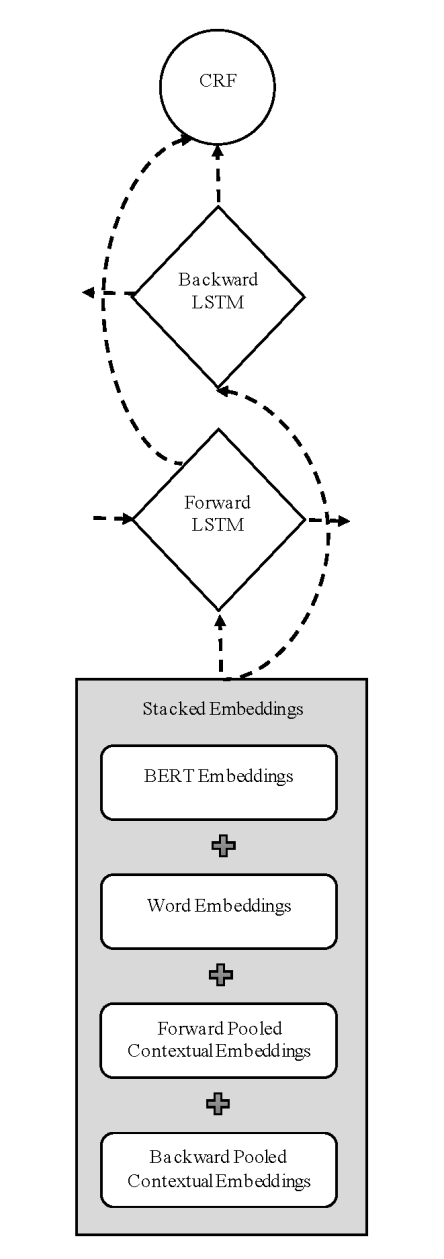
A Multi-Embeddings Approach Coupled with Deep Learning for Arabic Named Entity Recognition
Named Entity Recognition (NER) is an important task in many natural language processing applications. There are several studies that have focused on NER for the English language. However, there are some limitations when applying the current methodologies directly on the Arabic language text. Recent studies have shown the effectiveness of pooled contextual embedding representations and significant improvements in English NER tasks. This work investigates the performance of pooled contextual embeddings and bidirectional encoder representations from Transformers (BERT) model when used for NER on the Arabic language while addressing Arabic specific issues. The proposed method is an end-to-end deep learning model that utilizes a combination of pre-trained word embeddings, pooled contextual embeddings, and BERT model. Embeddings are then fed into bidirectional long-short term memory networks with a conditional random field. Different types of classical and contextual embeddings were experimented to pool for the best model. The proposed method achieves an F1 score of 77.62% on the AQMAR dataset, outperforming all previously published results of deep learning, and non-deep learning models on the same dataset. The presented results also surpass those of the wining system for the same task on the same data in the Topcoder website competition. © 2020 IEEE.