Breadcrumb
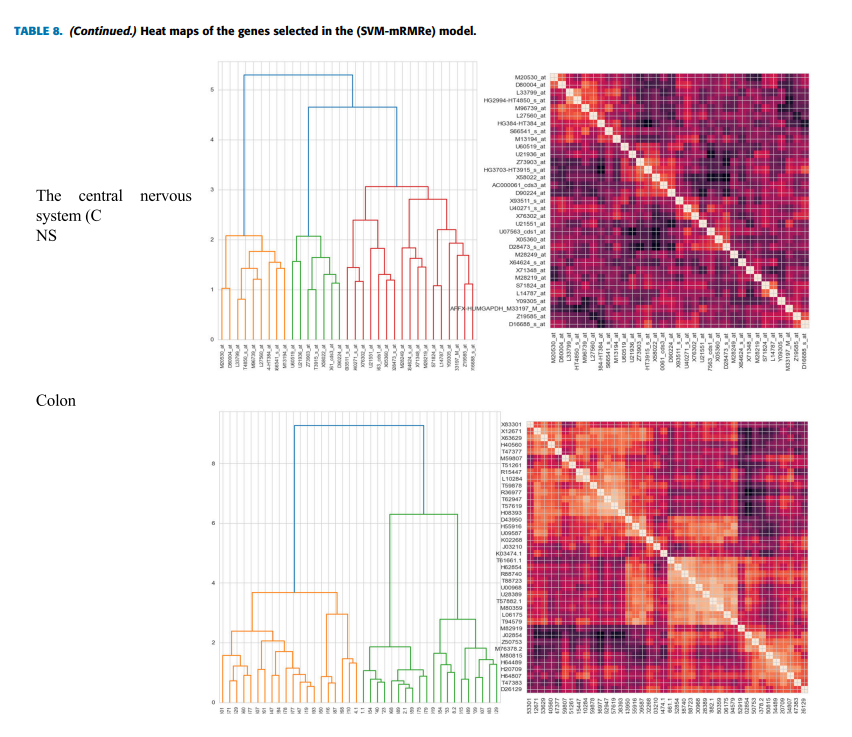
An Efficient SVM-Based Feature Selection Model for Cancer Classification Using High-Dimensional Microarray Data
Feature selection is critical in analyzing microarray data, which has many features (genes) or dimensions. However, with only a few samples the large search space and time consumed during their selection make selecting relevant and informative genes that improve classification performance a complex task. This paper proposed a hybrid model for gene selection known as (SVM-mRMRe), the proposed model provides a framework for combining filter-based, ensemble, and embedded methods to select the most relevant and informative genes from high-dimensional microarray data by fusing embedded SVM coefficients (features ranking) with ensemble mRMRe. Eight of the most commonly used microarray datasets for various types of cancer were used to evaluate the model. The selected subset feature is evaluated by four different types of classifiers: random forest (RF), multilayer perceptron (MLP), k-nearest neighbors (k-NN), and Support Vector Machine (SVM). The experimental results show that the proposed model reduces time consumption and dimensionality and improves the differentiation of cancer tissues from benign tissues. Furthermore, the selected genes for the brain cancer dataset are biologically interpreted, and it agrees with the findings of relevant biomedical studies and plays an important role in patient prognosis. © 2013 IEEE.