Breadcrumb
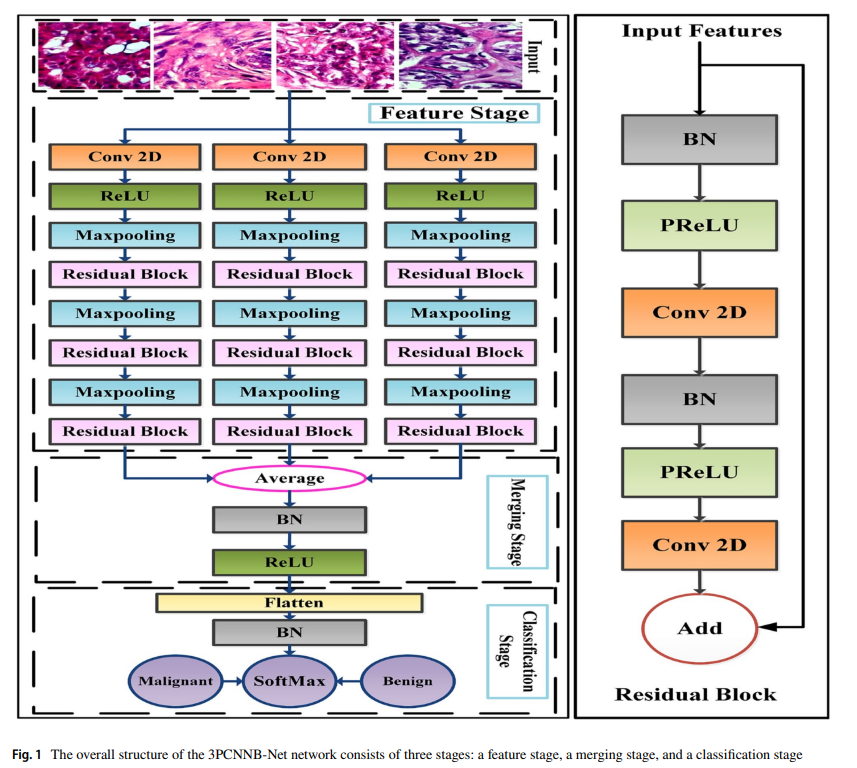
3PCNNB-Net: Three Parallel CNN Branches for Breast Cancer Classification Through Histopathological Images
Purpose: Diagnosis of breast tumors using histopathological imaging is considered a difficult task. Oncologists may have different opinions on how to use this imaging technique to diagnose tumors. This technique requires classification experience owing to the contrasting appearance caused by tissue preparation, staining processes, and disease heterogeneity. Cancerous breast tissues are classified into malignant and benign tumors according to cell diversity and density. Computer-aided diagnosis (CAD) helps oncologists improve breast tumor diagnosis efficiently and accurately while saving time for early diagnosis. Methods: Deep learning has begun to evolve in recent decades, and a convolutional neural network (CNN) is used to classify breast histopathological images. The proposed network of three parallel CNN branches (3PCNNB-Net) contains three stages. The first stage consists of three parallel CNN branches with deep residual blocks. In the second stage, we merged the three parallel branches to create a feature fusion path. Then, the fused features were classified in the third stage. The method presented was evaluated by experimenting with the BreakHis database for four magnificent factors. Results: The BreakHis database was used and implemented. It Contains 7909 images of breast tumor tissues taken from 82 patients. This method achieved 97.14% accuracy. The results were compared with the results of different techniques in the literature. Conclusion: This study demonstrated a new CNN model for automated breast cancer classification. The method presented successfully classified benign and malignant tumors. © 2021, Taiwanese Society of Biomedical Engineering.