Breadcrumb
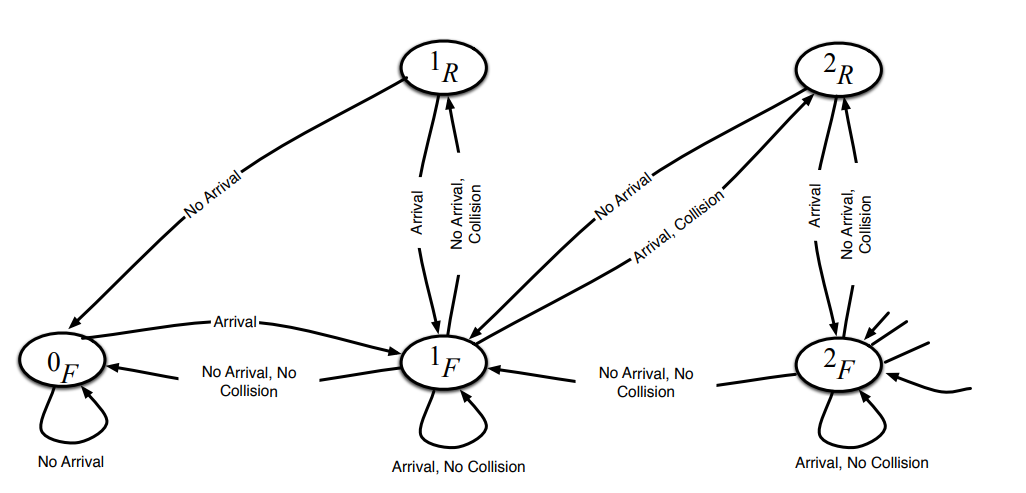
A reinforcement learning approach to ARQ feedback-based multiple access for cognitive radio networks
In this paper, we propose a reinforcement learning (RL) approach to design an access scheme for secondary users (SUs) in a cognitive radio (CR) network. In the proposed scheme, we introduce a deep Q-network to enable SUs to access the primary user (PU) channel based on their past experience and the history of the PU network's automatic repeat request (ARQ) feedback. In essence, SUs cooperate to avoid collisions with other SUs and, more importantly, with the PU network. Since SUs cannot observe the state of the PUs queues, they partially observe the system's state by listening to the PUs' ARQ packets. To model this system, a Partially Observable Markov Decision Process (POMDP) is adopted, and an RL deep Q-network is employed for the SUs to learn the best actions. A comparative study between the proposed scheme with baseline schemes from the literature is presented. We also compare the proposed scheme with the perfect sensing system (which constitutes an upper bound on the performance) and the system exploiting only the last ARQ feedback. Our results show that the proposed RL based access scheme yields comparable performance to the baseline ARQ-based access schemes, yet, with minimal knowledge about the environment compared to the baseline which assumes perfect knowledge of key system parameters, e.g., PUs arrival rates. On the contrary, our proposed scheme autonomously learns these parameters and, hence, dynamically adapts to their variation. © 2021 IEEE.