Breadcrumb
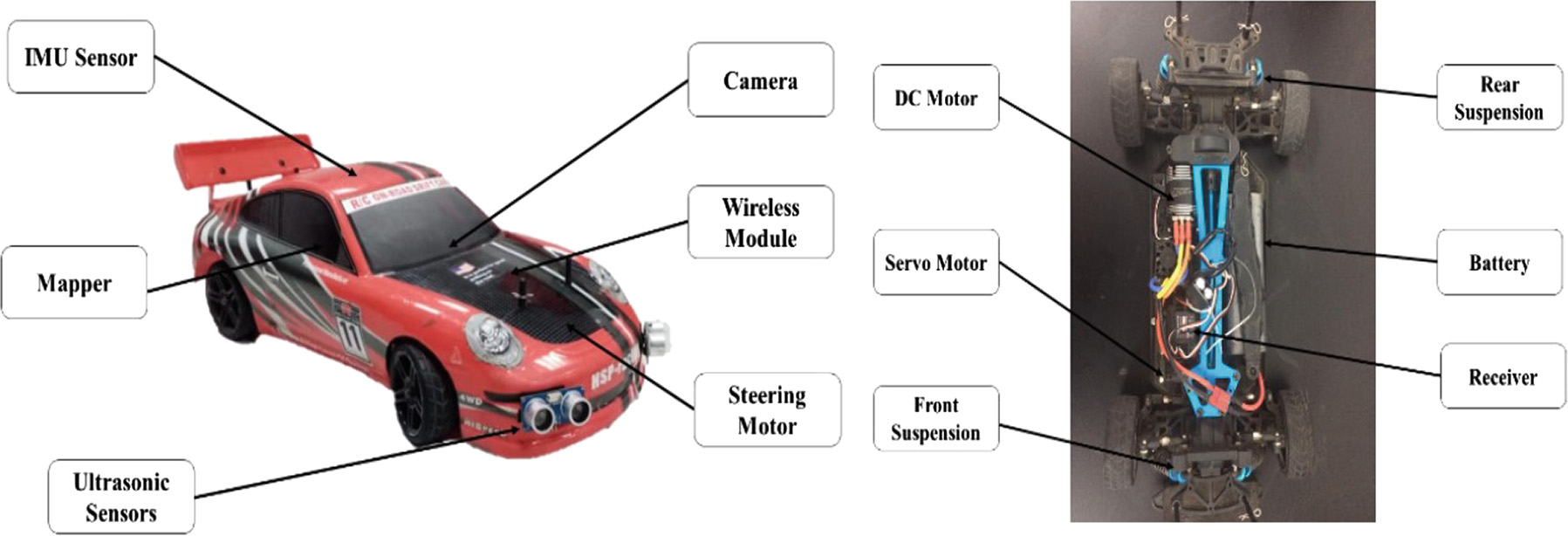
A Hybrid Deep Learning Based Autonomous Vehicle Navigation and Obstacles Avoidance
Technological revolution has reached all life activities starting from day planning reaching communication, entertainment, industry, and transportation. Each of previously mentioned categories get improved in a way making human life easier and safer. In the use of automatic control, several researches focused on automating vehicles’ systems to make driving easier and safer. The availability of autonomous vehicles will avoid accidents caused by taking a late decision or lack of driving experience in such situation. Approaching autonomous driving, an autonomous vehicle must be able to respond to the state of objects in the surrounding, be it stationary or in motion. This paper outlines the techniques which enable the car to become conscious of its immediate environment while it moves independently and to decide its next course of action to avoid obstacles. It investigates two approaches which are Neuro-Fuzzy System tuned by Particle Swarm Optimization (PSO) and Convolutional Neural Network (CNN) tuned by Adaptive Moment estimation (Adam). Such control can allow cars on roads to operate smoothly and, according to trained data, take quick accurate decisions. Results showed high performance of deep learning algorithms specially CNN with Adam; however, it needs more computational time than Neuro-Fuzzy system tuned with PSO. © 2020, Springer Nature Switzerland AG.